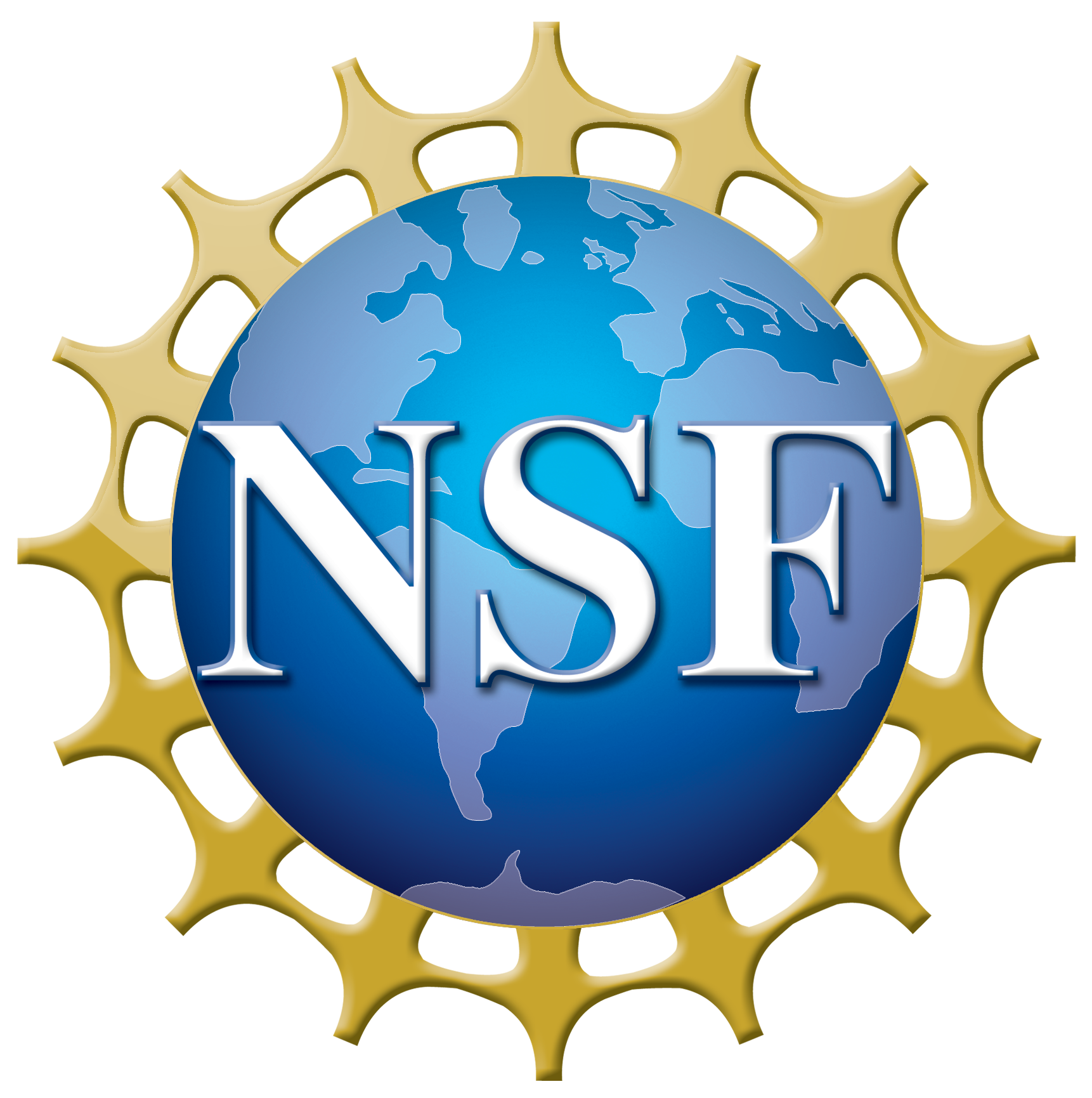
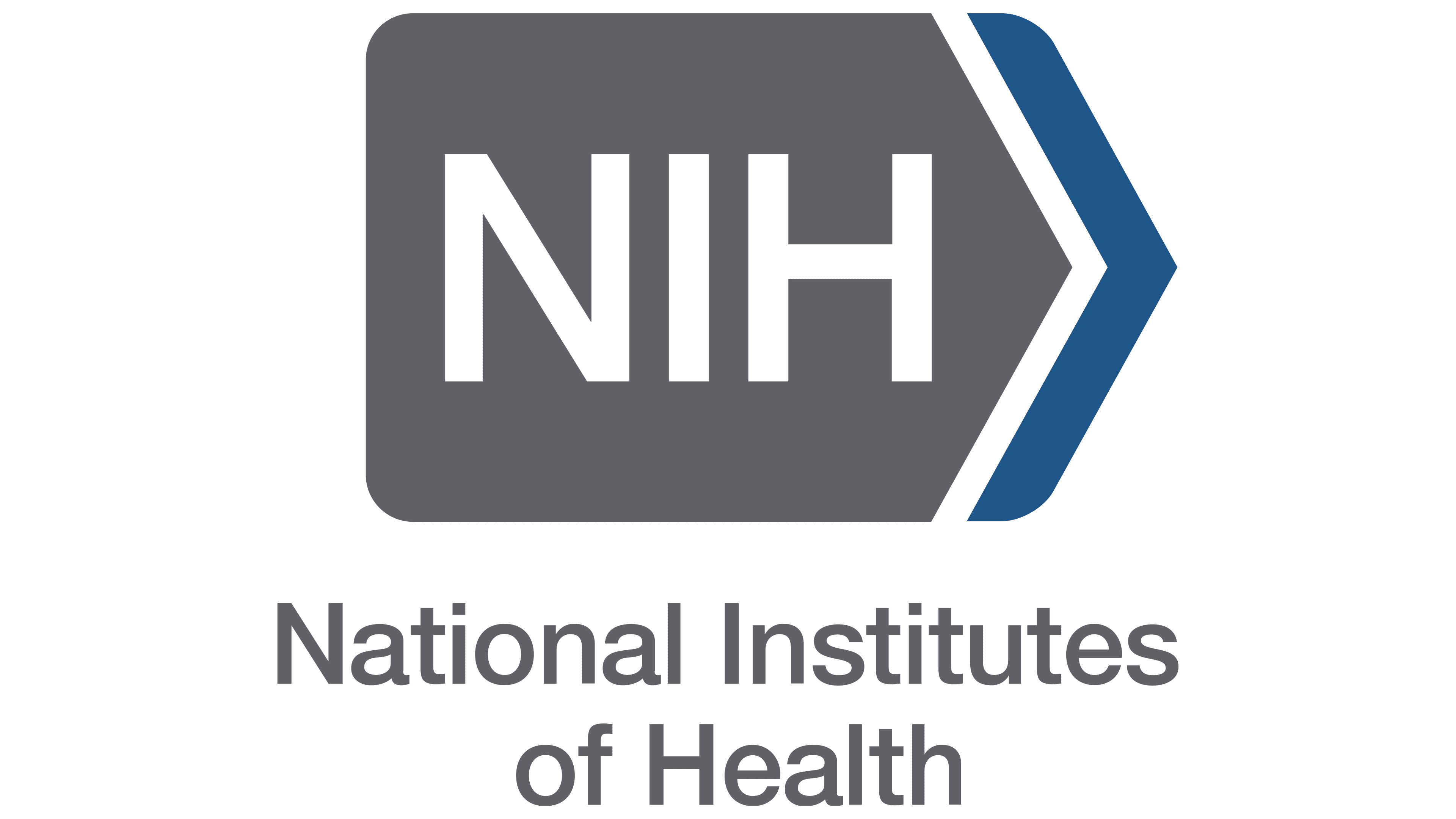
My research focuses on the development of statistical methods for analysis of modern high-dimensional biomedical data, and is at the intersection of applied, computational and theoretical statistics. I believe that challenging applied problems give rise to better statistical methodology and my primary goal is to aid the discovery of scientifically meaningful low-dimensional structures in high-dimensional data. I believe that collaboration plays a key role in achieving this goal and I enjoy working with both domain scientists and methodological researchers. My research has been supported by the National Science Foundation grants DMS-1712943, CAREER DMS-2044823, NIH R01HL172785 from NHLBI and recognized with a David P. Byar Young Investigator Award from the Biometrics section of the American Statistical Association.
Summary of methodological interests:
- high-dimensional data analysis (with the focus on sparsity regularization)
- multivariate analysis (dimension reduction methods such as PCA, CCA and LDA)
- data integration/multi-view data analysis
- statistical/machine learning
- computational statistics
Summary of application interests:
- multi-omics data analysis (e.g. gene expression, methylation, miRNA, etc.)
- microbiome data
- data from wearable devices (e.g. continuous glucose monitors and activity trackers)